Multi-Agent Simulation of Hydrogen Transition Issues
Background: The early transition phase in a possible future shift to a hydrogen-based transportation system will likely be a time of considerable uncertainty, when reasonable investors might hold widely differing expectations and employ a variety of business strategies to pursue divergent company goals. Some may anticipate rapid technology development, active government support and strong market growth; others may be less optimistic.
In collaboration with partners, such as RCF Consulting LLC, Ford Motor Company, BP, and others, the Center for Energy, Environmental, and Economic Systems Analysis (CEEESA) is using agent-based modeling and simulation (ABMS) techniques to analyze this early transition phase. ABMS is a micro-simulation technique that facilitates representation of heterogeneity and is well suited to applying sophisticated economic and behavioral models in an environment involving actors with widely differing characteristics and goals and limited foresight.
As opposed to conventional approaches, ABMS does not enforce a global optimum solution. Rather, it incorporates different objective functions (goals) for different agents and allows for varied reactions to unmet expectations and exogenous events, different learning from the emerging economic environment, and diverse responses based on agent characteristics.
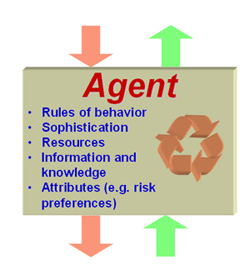 |
Methodology: Conventional methods to address technology introduction and deployment issues rely on developing computer simulations to project how a new product will be accepted in the marketplace. These tools rely on conventional optimization approaches, equilibrium analysis, and other analytical techniques. These conventional approaches are, however, less suitable for evaluating the manner in which the hydrogen infrastructure might evolve. There are far too many complex interactions among the many potential participating entities to be captured with these techniques. Further, the long-term configuration of the hydrogen infrastructure is not the only issue of interest. Understanding the alternative evolutionary stages that the infrastructure may pass through is critical to identifying the conditions under which it will succeed or fail. ABMS as applied to hydrogen transition analysis has the following key characteristics:
- Simulates the behaviors and interactions of a large number of individuals (agents) and studies the macro-scale consequences of these interactions: current model runs are for about 7,000-70,000 agents
- Represents diversity of actors: different tastes, resources, strategies, risk preferences, etc.
- Represents independent decision making: each stakeholder maximizes its own objective or utility function
- Decisions are made under uncertainty and based on imperfect knowledge
- Stakeholders learn and adapt
Current Model Implementation: In the current model implementation we use the road topology of a real metropolitan area, the Los Angeles basin. The driver agents vary in their income and live and work in locations spread across the LA area based on demographic data. They have attributes such as “greenness” – their willingness to pay more or suffer more inconvenience to operate a vehicle that has desirable environmental characteristics.
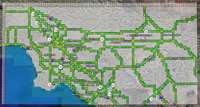 |
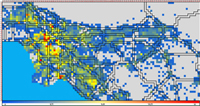 |
H2CAS Road Grid
|
H2CAS Number of Homes
|
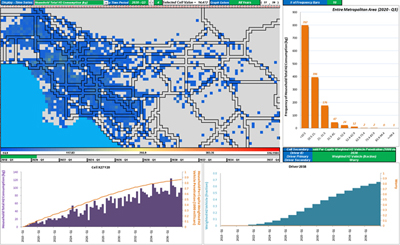 |
H2CAS Results Interface |
The driver agents are influenced in their purchase decisions not only by their personal experience and a general “belief space” reflecting the attitude of society in general towards hydrogen, but also by interactions with their peers. The purchase decision is implemented in the form of a utility function which computes the sum of all adoption incentives less the disincentives of inconvenience and worry about running out of fuel. We take advantage of Repast Simphony’s networking capabilities to set up relationships between the agents based on their home neighborhoods, their places of work, and common characteristics. We are currently experimenting with Moore neighborhoods but may eventually extend this to include full social networking capabilities in the future.
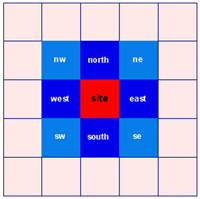 |
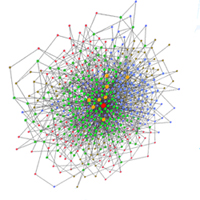 |
Example H2CAS Moore Neighborhood |
Example H2CAS Social Network |
In summary, our model includes endogenous vehicle adoption and fuel purchases on a GIS platform. Our driver or consumer agents decide to buy hydrogen vehicles and when and where to refuel based on their assessment of fuel availability and other factors, including
- The need to refuel at inconvenient places/times and the risk (worry) of running out of fuel,
- The price differentials of vehicle and fuel (H2 versus gasoline),
- Their knowledge about H2 vehicles,
- The influence of their neighbors and colleagues,
- Their attitudes toward new technologies, and
- Their socioeconomic characteristics (e.g., income, location, miles driven).
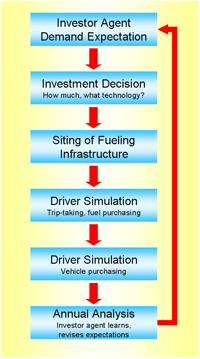 |
The investor agents in the model decide the type of supply infrastructure, and how much and where to locate it. These investment decisions are made with imperfect knowledge of driver vehicle purchase and fueling behavior, the expected penetration of hydrogen, the plans of competitors, and the total demand for fuel. Thus, investors need to develop expectations they can use to base their decisions on. We model investors as agents that are trying to do the best they can, given the uncertainties they face. Our investors may make non-optimal decisions and may learn from their experience. In a sense, our investors are satisficing rather than optimizing.
The investor's knowledge is limited to certain observations on what has happened in the past (such as fuel sales in particular locations) with some crude assumptions on what they might be in the future. With this limited knowledge, the investor agents develop their demand expectations on which they base their investment decisions. As the driver agents respond to the roll-out of the supply infrastructure by purchasing H2 vehicles and fuel, the investor receives feedback on the actual demand realization. He uses this in an ex-post analysis to revise his expectations and adjust his future investment plans.
Rather than having single-value expectations, our investor agents have subjective probability density functions of sales. The investor uses rules of thumb to determine whether and where to locate fueling stations. He does this by trying to maximize his utility function. The investor’s approach to risk is to balance expected profits with the risk of bankruptcy, with greater weight put on the bankruptcy outcome the more risk averse the investor is. We distinguish between different types of investor agents, from risk prone to risk averse, with different relative risk aversion coefficients.
For learning over time, as the investor acquires more and more knowledge about the reality of hydrogen fuel sales, a simple form of Bayesian learning is used.
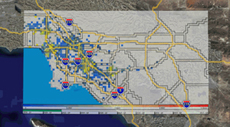 |
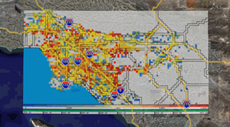 |
H2CAS Simulated Vehicle Adoption Snapshot 1 |
H2CAS Simulated Vehicle Adoption Snapshot 2 |
|
H2CAS Animation of Vehicle Adoption over Time |
This ongoing work leverages several existing activities including the following:
(a) The Center for Complex Adaptive Systems has been working for the past four years on the development of computer simulation toolkits, ABMS modeling techniques, and software engineering methods to use in agent-based models. These have resulted in the development of ABMS capabilities that have been applied to several other domains including deregulated electricity markets, counterterrorism analysis, and retail sales. The experience gained in these programs will significantly enhance the ability to use the ABMS approach on the hydrogen infrastructure problem.
(b) The Center for Energy, Environmental, and Economic Systems Analysis has developed numerous energy system analysis tools that have been applied to domestic and international energy system issues. This work will provide the detailed background needed to structure the hydrogen infrastructure configuration alternatives, to assemble the energy system technical and economic data needed for the modeling effort, and to construct the conventional model of the energy infrastructure.
(c) The Center for Transportation Research has developed several models of consumer choice, vehicle cost, transportation demand, and hydrogen infrastructure. These will provide key insights into consumer and manufacturer decision behavior and the configuration of alternative hydrogen infrastructures, as well as a suite of models to estimate hydrogen fuel demand, investment requirements, and fuel cost for the conventional model of the energy infrastructure.
Additional Resources: For more information on this modeling activity, use the links below:
For more information, contact contact CEEESA
|